Since December 2018, we are organizing along with Liubov Tupikina (Bell Labs) a network seminar series at LPI Paris. Talks showcase the use of network science and hypergraphs in a wide range of disciplines, from physics to mathematics, biology, social sciences or neurosciences, and are intended for a broad, interdisciplinary audience.
Below you can find the past speakers and recordings. Please contact us if you know someone working with networks who would be a great speaker for this event! Talks are 45 min + 15 min questions, and are usually held on Thursdays at 5pm in hybrid form on Zoom and at LPI Paris. Recordings are also available on Youtube.
For description of upcoming events and the latest updates please subscribe to our Mailing list.
Past events:
- 16 Apr 2024 – Nicholas Landry (University of Vermont) – Higher-order structure is more complex than current measures and models
- 28 March 2024 – Jean-Gabriel Young (University of Vermont) – What can we learn from low-dimensions representations of networks?
- 1 Feb 2024 – Levin Brinkmann (Max Planck Institute) – Exploring the Evolution of Artistic Styles Using Generative AI
- 5 October 2023 – Quintino Francesco Lotito (University of Trento) – Higher-Order Networks and Motif Analysis in Hypergraphs
- 27 April 2023 – Dmitry Kobak (Tübingen University) – Contrastive and neighbor embedding methods for data visualisation
- 15 December 2022 – Alexander Belikov (University of Chicago) – Quantifying Scientific Discovery to Improve the Knowledge of Facts
- 17 November 2022 – Mike Tamm (Tallinn University) – Nearest-neighbour network in hyperbolic space: a simple model with applications to recommendation systems.
- 3 November 2022 – Eszter Bokányi (POPNET) – The anatomy of a population-scale social network
- 16 June 2022 – Frank Takes (Leiden University) – Population-scale Social Network Analysis
- 12 May 2022 – Bernardo Monechi (Sony CSL) – Kreyon City: Finding successful strategies in a complex urban sustainability game
- 28 April 2022 – Giona Casiraghi (ETH Zurich) – Statistical Modelling of Multi-Edge Networks
- 16 Feb 2022 – Marc Barthelemy (IPhT) – Modelling the population evolution of cities
- 25 Nov 2021 – Renaud Lambiotte (Oxford) – Order and Disorder in Network Science
- 16 Sept 2021 – Laura Cantini (CNRS, ENS) – Network-guided integration of multi-omics data: towards a comprehensive view of cancer
- 8 July 2021 – Marc Timme (TU Dresden) – Inverse problems and data-driven modeling for multi-dimensional dynamical systems — from biology to mobility
- 3 June 2021 – Gerardo Iñiguez (CEU) – Dynamics of cascades on burstiness-controlled temporal networks
- 21 Jan 2021 – Jürgen Kurths (Humboldt University) – Quantifying Stability in Deterministic and Stochastic Complex Networks and its Application to Power Grids
- 10 Dec 2020 – Tiago P. Peixoto (CEU) – Network reconstruction from indirect observations
- 19 Nov 2020 – Michele Starnini (ISI Foundation) – Emergence of echo chambers and polarization dynamics in social networks
- 12 Nov 2020 – Geoffroy Berthelot (INSEP) – Pseudo-Darwinian network evolution
- 5 Nov 2020 – Massimo Stella (University of Exeter) – Cognitive network science as a proxy for accessing key ideas and emotions in people’s minds
- 8 Oct 2020 – Elisa Omodei (World Food Programme’s Research) – Who, where, why: non-traditional data and predictive analytics to map socio-economic vulnerabilities
- 9 July 2020 – Petter Holme (Tokyo Tech) – Freedom of choice adds value to public goods
- 28 May 2020 – Luca Aiello (Bell Labs, Cambridge) – Ten Dimensions of Social Relationships
- 27 Feb 2020 – Jean-philippe Cointet (Sciences Po Media Lab) – The structure of the French media space and the Yellow vest movement
- 28 Nov 2019 – Blaise Delmotte (CNRS, LadHyX, Ecole Polytechnique) – Leveraging Collective Effects in Active Fluids
- 17 Oct 2019 – Brennan Klein (Network Science Institute, Northeastern University) – The structure is the story: How the right representation can bring forth new theories in complex systems
- 9 Oct 2019 – Ray Rivers (Imperial College) – Myth and Math: A network approach to Atlantis
- 3 Oct 2019 – Elizabeth Vergu (INRA) – Data analysis and model-based indicators of nodes criticality for epidemic spread and control on a cattle trade network
- 26 Sept 2019 – Vincent Hakim (CNRS, ENS) – Puzzles about long term memory and neuronal learning
- 12 Sept 2019 – Federico Battiston (CEU) – New emergent behavior in generalized networks
- 14 July 2019 – Jennifer Dunne (Santa Fe Institute) – Examining human-centered ecological networks across the world
- 27 June 2019 – Daniele Marinazzo (Ghent University, Belgium) – Synergy and Redundancy: a Network Perspective
- 20 June 2019 – Charles Baroud (Ecole Polytechnique, Pasteur, France)
- 13 June 2019 – Henry C. W. Price (Imperial College, London) – From Local to Global: Nested Interaction and Community in Late Bronze Age Crete. A network approach.
- 6 June 2019 – Janet Bennion (Vermont University) – Polyamory in Paris: A Social Network Theory Application
- 23 May 2019 – Guillaume Dumas (Pasteur Institute) – Networks and Machine Learning in Biomedical Research: from Generative Models in Social Neurosciences to Multi-Scale Priors in Precision Medicine
- 18 April 2019 – Alexey Medvedev (Université de Namur and Université catholique de Louvain) – Predicting discussion dynamics in online forums using Hawkes processes: case study of Reddit
- 28 Feb 2019 – Chiara Poletto (INSERM) – Accounting for variable and heterogeneous human behaviour in the assessment of an epidemic
- 6 Dec 2018 – Christian Vestergaard (Pasteur Institute) – Randomized reference models for temporal networks
16 Apr 2024 – Nicholas Landry (University of Vermont) – Higher-order structure is more complex than current measures and models

Modelling heterogeneous contact patterns between individuals as a pairwise network, where all interactions occur between two individuals, has yielded valuable insights into the structure and the dynamical behavior of complex systems. Higher-order networks relax this pairwise assumption and represent group interactions of arbitrary size, which can more closely represent the rich structure and dynamics of empirical systems. In contrast to pairwise networks, interactions in higher-order networks can overlap, differ in size, and include one another. We show that there are critical limitations in the measurement and modeling of higher-order networks. First, although researchers often assume that the structure of a higher-order system is consistent across all scales of interaction, connection patterns of individuals or entities in empirical systems are often stratified by interaction size. We address this limitation by introducing an approach for filtering higher-order datasets. Second, traditional modelling approaches to higher-order networks tend to either not consider inclusion at all (e.g., hypergraph models) or explicitly assume perfect and complete inclusion (e.g., simplicial complex models). We show that, contrary to current modelling practice, empirically observed systems rarely lie at either end of this spectrum and that generative models fitted to empirical datasets rarely capture their inclusion structure.
Nicholas Landry is the TGIR Postdoctoral Research Fellow at the University of Vermont. His research expertise is broadly the study of dynamics on complex systems, especially the spread of contagion on interaction networks involving group (higher-order) interactions.
28 March 2024 – Jean-Gabriel Young (University of Vermont) – What can we learn from low-dimensions representations of networks?
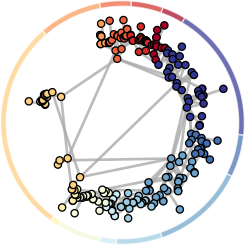
Abstract: Machine learning systems now routinely use embeddings in thousands of dimensions to extract patterns from large-scale network data. Should we embrace this data revolution and let go of simpler network theories—S1 models, Bradley-Terry models, and so on?
In this talk, I will argue that low-dimensional embedding can reveal powerful yet interpretable network patterns and thus have a place in any modern data science stack.
I will illustrate this point through a number of stories about social hierarchies and decision-making.
Bio: Jean-Gabriel Young is an Assistant Professor of Mathematics and Statistics at The University of Vermont, VT, USA, where he co-directs the joint lab. His research focuses on the intersection of statistical inference, epidemiology, and complex systems. Previously, he was a James S. McDonnell Foundation Fellow at the Center for the Study of Complex Systems at the University of Michigan, mentored by Prof. Mark Newman. He obtained his PhD in Physics from Université Laval, under the guidance of Prof. Louis J. Dubé and Prof. Patrick Desrosiers.
1 Feb 2024 – Levin Brinkmann (Max Planck Institute) – Exploring the Evolution of Artistic Styles Using Generative AI

Abstract: Understanding the evolution of human creative expression is central to art history, and instrumental to the progress of algorithmic creativity. Recent advances in generative AI, such as Stable Diffusion, Midjourney, and DALL-E, show great promise in generating detailed images based on textual prompts blending visual concepts and art styles. However, whether these models can produce truly novel outputs beyond recombination remains unclear. Measuring creativity and cultural progress in subjective domains like the arts is challenging, but generative AI can help deconstruct art into distinct concepts, such as style, content, and composition, and measure their similarity. In this ongoing work, we propose a method to measure the similarity of visual concepts and thereby the cultural evolution of artist styles. We also present a simple influence model to represent the cultural processes, discuss the convex hull in embedding space as a measure of humanity’s commutative cultural repertoire, and discuss the potential of generative AI to explore new, unseen art styles.
Levin Brinkmann, a Ph.D. candidate at the Max Planck Institute for Human Development under the supervision of Iyad Rahwan, blends complex systems, machine learning, and experimental social science to study human-AI hybrid systems. His non-academic work includes applying contrastive learning to enhance the work of professional stylists. During his Ph.D., he conducts experiments on behavior transmission between deep Q-learning agents and humans, as well as on collaborative exploration of visual concepts using diffusion models. Levin’s work is driven by his passion for integrating insights from collective intelligence and cultural evolution into AI, aiming to develop prosocial and innovative AI ecosystems.
5 October 2023 – Quintino Francesco Lotito (University of Trento) – Higher-Order Networks and Motif Analysis in Hypergraphs
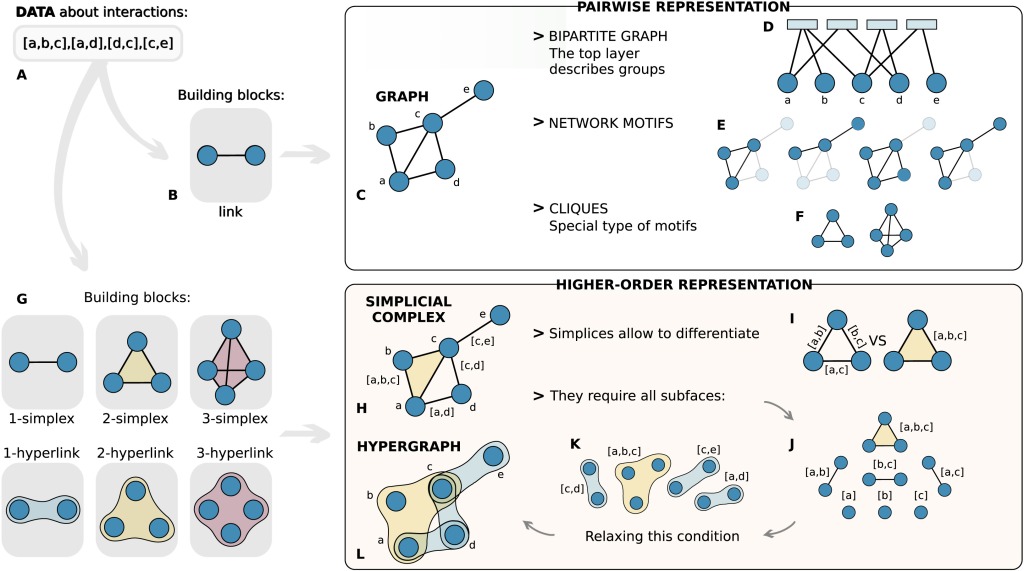
Abstract: Over the last two decades, networks have emerged as a powerful tool to analyze the complex topology of interacting systems. From social networks to the brain, several systems have been represented as a collection of nodes and links, encoding dyadic interactions among pairs of units. Yet, growing empirical evidence is now suggesting that a large number of such interactions are not limited to pairs, but rather occur in larger groups. In this seminar, we will discuss how more sophisticated mathematical frameworks such as the hypergraphs can enhance our modeling capabilities for systems involving higher-order interactions. We will see that dealing with such complex structures requires new algorithms to cope with more computationally difficult problems, and new tools and generalizations of classic network ideas to fully exploit the improvements in the expressive power. In the last part of the talk, we will focus on the specific problem of higher-order motif analysis. Higher-order network motifs are defined as statistically over-expressed connected subgraphs of a given number of nodes, which can be connected by higher-order interactions of arbitrary order. We will show how they are able to characterize the local structure of hypergraphs and extract fingerprints at the network microscale of higher-order real-world systems. Moreover, we will discuss the problem from an algorithmic perspective, investigating also some real-world applications. Finally, we will talk about open challenges and possible future directions.
Bio: Quintino Francesco Lotito is a PhD student in Computer Science at the University of Trento in Italy. His research focuses on the development of statistical methods and efficient algorithms to analyze network data. In particular, he is interested in characterizing the structural organization at multiple scales of real-world complex systems with group interactions, from social to biological systems.
👉🏼 Python library for higher-order network analysis: https://arxiv.org/pdf/2303.15356.pdf
👉🏼 Higher-order network motif analysis in hypergraphs: https://www.nature.com/articles/s42005-022-00858-7
👉🏼 Review article about higher-order networks: https://www.sciencedirect.com/science/article/pii/S0370157320302489
27 April 2023 – Dmitry Kobak (Tübingen University) – Contrastive and neighbor embedding methods for data visualisation

Abstract: In recent years, neighbor embedding methods like t-SNE and UMAP have become widely used across several application fields, in particular in single-cell biology. They are also widely used for visualising large collections of documents and/or images used to train modern deep learning architectures such as large language models or diffusion models. Given this academic and public attention, it is very important to understand possibilities, shortcomings, and trade-offs of neighbor embedding methods. I am going to present our recent work on the attraction-repulsion spectrum of neighbor embeddings and the involved trade-offs. I am also going to explain how neighbor embeddings are related to contrastive learning, a popular framework for self-supervised learning of image data. This will lead to our recent work on contrastive visualizations of image datasets. In the second part of the talk, I will present our ongoing work on visualization of scientific literature, in particular biomedical research papers from the PubMed library.
Bio: Dmitry Kobak is a research scientist and a group leader in the Berens lab at Tübingen University, Germany. He is interested in unsupervised and self-supervised learning, in particular contrastive learning, manifold learning, and dimensionality reduction for 2D visualization of biological datasets.
👉🏼 More information about Dimitrii and some work he made public https://twitter.com/hippopedoid
👉🏼 Some of his publications: https://www.biorxiv.org/content/10.1101/2023.04.10.536208
15 December 2022 – Alexander Belikov (University of Chicago) – Quantifying Scientific Discovery to Improve the Knowledge of Facts
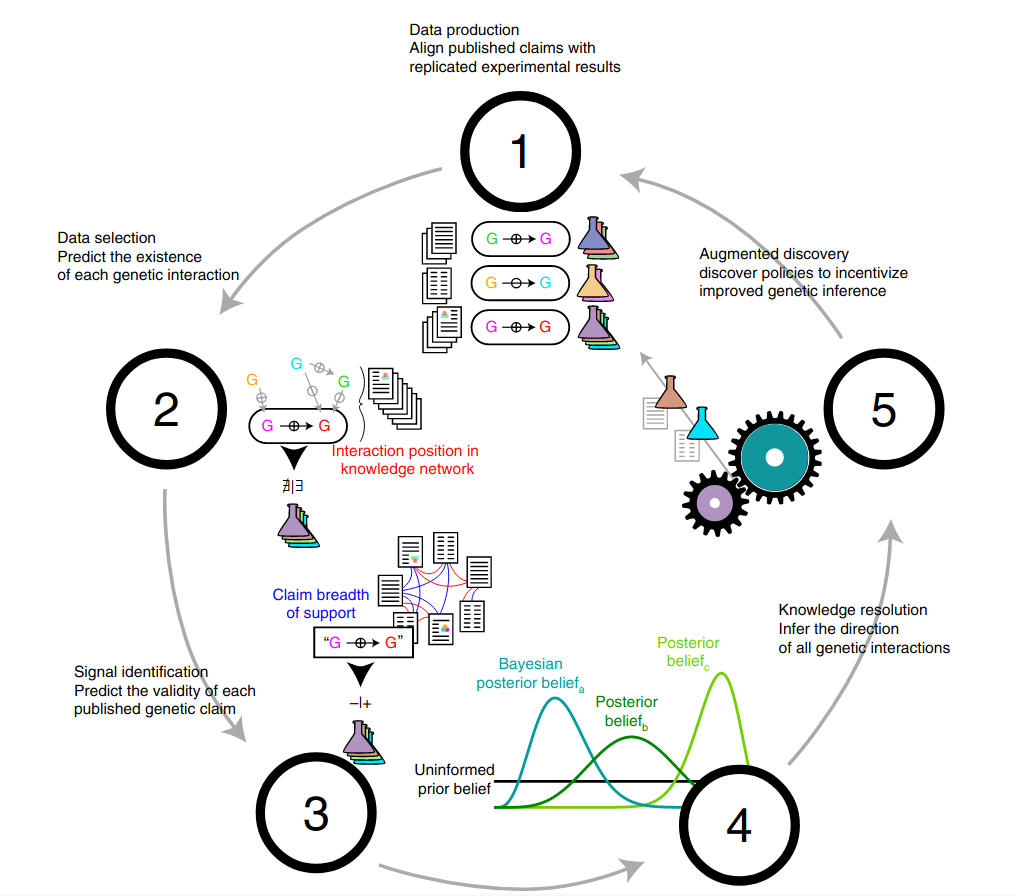
Abstract: The ever-increasing amount of published academic results poses a challenge in interpretation and validation of these publications and rendering them to scientific facts. Despite the apparent lack of alignment between published claims and established facts, accounting for network structure enables predictive models that can assess the validity of published claims. Using pre-trained models on simulated alternative attention and local clustering distributions (which translates to modifications of funding policies) of academic publication we show that the overall knowledge of facts may be dramatically improved. We conclude by a discussion of applications of our methodology to other domains.
Bio: Sasha (Alexander) Belikov started his career as a physicist with contributions in condensed matter and dark matter physics. After switching gears to become a quantitative researcher in finance for 2 years, he then did a postdoc in computational sociology at the Knowledge Lab at the University of Chicago. In the past 3 years, while leading the data science team at a Parisian start-up Hello Watt, he has remained involved in modeling scientific processes and the development of tools thereof.
17 November 2022 – Mike Tamm (Tallinn University) – Nearest-neighbour network in hyperbolic space: a simple model with applications to recommendation systems.

Abstract: Reference and recommendation networks are ubiquitous: encyclopedia articles and scientific papers refer to each other, people recommend each other books, films and music, online shops are full of “people who like this also like that” recommendations. Such networks are substantially asymmetric: the rules according to which a node becomes a source are different from those according to which it becomes a target. Often, the number of recommendations given is effectively bounded, while the number of times a node is recommended (in-degree) is unlimited and has a wide distribution.
Here we suggest a relatively simple model of a directed network with asymmetric degree distribution: narrow distribution of out degree and (generally speaking) wide distribution of the in degree, and study its structural properties. Our model belongs to the hyperbolic class and is a direct generalization of nearest-neighbor models studied extensively for the case of Euclidean metric spaces.
Consider a disk on a hyperbolic space, put a large number of points onto the disk uniformly at random, and then connect each point by directed links to a fixed number m of its nearest neighbors. We study the properties of resulting networks in the limit when the area of the disk diverges while the dimensionless density of the points (measured in the space curvature units) remains constant. We show that the resulting networks consist of two distinct parts, a central core where the in-degree has an approximately Poissonian distribution with average m, and peripheral part, where the average in-degree is position-dependent and increases exponentially with increasing distance from the periphery of the disk. The distribution of nodes between core and periphery is controlled by the dimensionless density of the nodes: in high-density networks the core dominates, while the low-density ones are dominated by the periphery.
The resulting overall in-degree distribution is a truncated power law with exponent -3, the width of the power-law region depends on the density, in the limit of low density the distribution becomes a true power law. Notably, the average in-degree of the network remains finite and equal to m in the thermodynamic limit.
We calculate additional structural properties of the network, such as the fraction of bidirectional links, and show how it can be regulated by introducing an additional temperature-like parameter governing the probability of link formation.
Bio: Mike Tamm is ERA Chair for Cultural Data Analytics, School of Digital Technologies, Tallinn University, Tallinn, Estonia
3 November 2022 – Eszter Bokányi (POPNET) – The anatomy of a population-scale social network

Abstract: Common large-scale approaches to inferring social structure make use of digital traces such as online social networks or mobile communication data. However, these networks are often agnostic of node and edge representativity and type. This talk investigates the structure of a social network sourced from administrative registers for an entire population based on family, household, work, school, and next-door neighbor relations, alongside rich demographic node attributes. We revisit three of the most common concepts in social network analysis: degree, closure and distance. We find that observed degrees are the result of a combination of degree distributions in various layers, disqualifying common explanatory mechanisms such as preferential attachment. Low node-to-node distances are realized through particular edge types that shortcut paths in already clustered areas. Measuring closure across layers shows how we can realistically capture the extent to which people have closed or open network opportunity structures. Finally, we highlight how people’s network structure varies greatly along demographic axes such as age, income and level of education. This shows that understanding of both the type of edge and the part of the population that is considered is of great importance. Therefore, leveraging register data to capture the social structure of a complete population is one of the most fruitful ways forward to obtain actionable insights and ultimately evidence-based policies.
Bio: Eszter Bokányi is a research postdoc in the POPNET project investigating a population-scale social network of the Netherlands. She has a background in physics, and graduated with a PhD from statistical physics at Eötvös Loránd University in Budapest. The topic of her thesis was already rather connected to computational social science, having investigated a huge database of geolocated Twitter messages.
Nowadays, she is still mostly interested in spatial social networks and their connection to human mobility, as well as dynamical phenomena such as the spreading of innovations over these social networks.
16 June 2022 – Frank Takes (Leiden University) – Population-scale Social Network Analysis
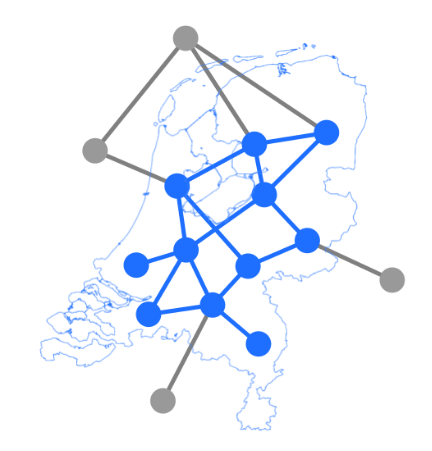
Abstract: This talk considers responsibly anonymized population-scale social network data on all 17 million inhabitants of the Netherlands. The data stems from country-wide administrative register data, and has the potential to shed new light on contemporary social scientific problems such as segregation, inequality, loneliness and poverty. The talk discusses how the formal links (family, household, work, school and neighbor ties) in this social network require one to critically rethink network science concepts such as the unit of analysis, measurement errors effects and the boundary specification problem. Moreover, it allows us to in a unique way revisit the well-known concept of closure and the small-world phenomenon in a population-scale social network context. The talk furthermore presents initial findings on the relation between the network structure and spatial distribution of the population.
Bio: Frank Takes is an associate professor at LIACS, the computer science and artificial intelligence department of Leiden University. His research interest is in network science, mainly dealing with methods and algorithms for knowledge discovery from (social) network data, covering applications in economics, science studies and computational social science. He supervises bachelor, master and PhD students, and teaches courses on competitive programming and social network analysis.Currently, he is the head of the Leiden Computational Network Science research group, academic co-director of the Dutch POPNET research platform for population-scale social network analysis and co-chair of the Dutch Network Science Society.
Webpage: https://franktakes.nl/
12 May 2022 – Bernardo Monechi (Sony CSL) – Kreyon City: Finding successful strategies in a complex urban sustainability game
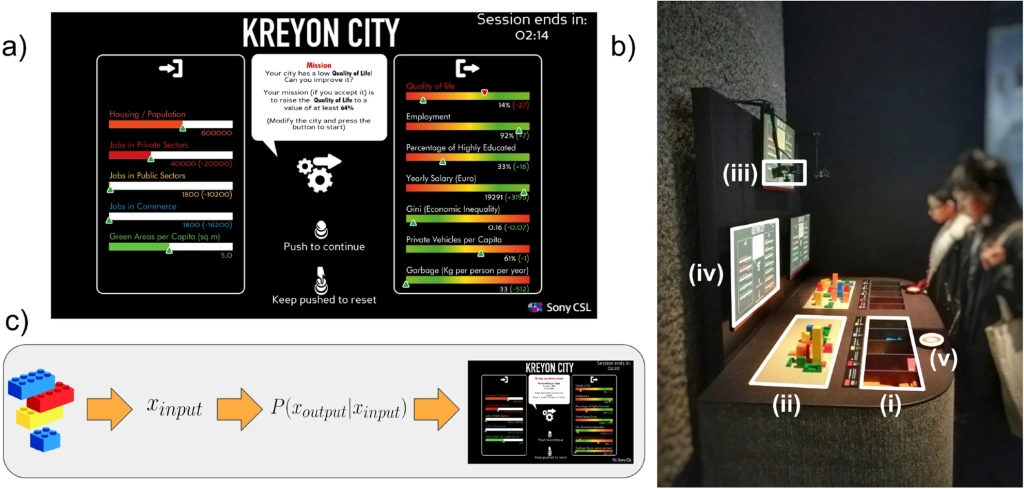
Abstract: The adverse effects of unsustainable behaviors on human society are leading to an increasingly urgent and critical need to change policies and practices worldwide. This requires that citizens become informed and engaged in participatory governance and measures leading to sustainable futures. Citizens’ understanding of the inherent complexity of sustainable systems is a necessary (though generally not sufficient) ingredient for them to understand controversial public policies and maintain the core principles of democratic societies. In this work, we present a novel, open-ended experiment where individuals had the opportunity to solve model urban sustainability problems in a purposeful game. Participants were challenged to interact with familiar LEGO blocks representing elements in a complex generative urban economic indicators model. Players seek to find a specific urban configuration satisfying particular sustainability requirements. We show that, despite the intrinsic complexity and non-linearity of the problems, participants’ ability to make counter-intuitive actions helps them find suitable solutions. Moreover, we show that through successive iterations of the experiment, participants can overcome the difficulties linked to non-linearity and increase the probability of finding the correct solution to the problem. We contend that this kind of what-if platforms could have a crucial role in future approaches to sustainable development goals.
Bio: Bernardo Monechi is a Researcher at Sony Computer Science Laboratories. He works in the Sustainable Cities unit, focused on understanding Urban Dynamics using Machine Learning, Statistical Physics, and Data-driven approaches. He is also involved in art-science interaction projects by bringing scientific content into museums or taking part in the artistic process with scientific tools.
28 April 2022 – Giona Casiraghi (ETH Zurich) – Statistical Modelling of Multi-Edge Networks
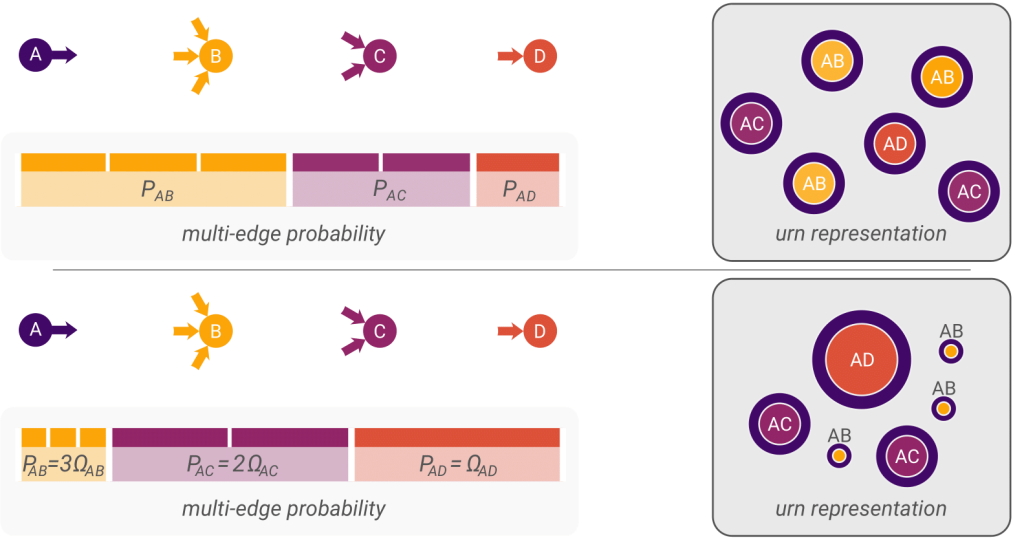
Abstract: A fundamental issue of network data science is the ability to discern observed features that can be expected at random from those beyond such expectations. Network models play a crucial role there. They allow us to test hypotheses and understand the mechanisms underlying observed phenomena in complex systems. Today, available network data provides a rich collection of information about nodes and their interactions. Between a pair of nodes, there could be a large number of different types of interactions, signalling the existence, or the absence, of relevant relations. In contrast, standard network models often focus on simple, unweighted graphs, unable to deal with repeated edges between nodes. Addressing this issue, here I will introduce the generalised hypergeometric ensemble of random graphs (gHypEG). By mapping the classical configuration model to an urn problem, GHypEG provides a flexible modelling framework for rich multi-edge network data. I will further show how different statistical hypotheses can be encoded and tested, highlighting how to overcome the challenges of modelling multi-edge networks.
Bio: Dr. Giona Casiraghi is a senior researcher in the Chair of Systems Design at ETH Zurich. He works on two tightly connected topics – Resilience of Social Organisations and Statistical Models for Network Data. Part of his work revolves around the generalised hypergeometric ensemble of random graphs, gHypEG for short.
Webpage: https://www.sg.ethz.ch/team/giona_casiraghi/
16 Feb 2022 – Marc Barthelemy (IPhT) – Modelling the population evolution of cities
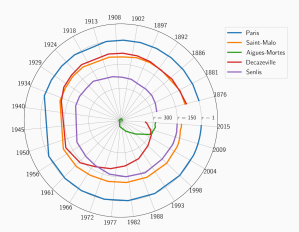
Abstract: Modelling the population evolution of cities is at the core of urban science. The most fundamental problem is to understand the hierarchical organization of city population and the statistical occurrence of megacities. This was first thought to be described by a universal principle known as Zipf’s law. However, the validity of this model has been challenged by recent empirical studies. In addition, we also observe empirically the relative frequent rise and falls of cities. I will present here a model able to explain and to reconciliate these various aspects of urban dynamics. More precisely, I will discuss a stochastic equation recently proposed for modelling population growth in cities, constructed from an empirical analysis of recent datasets (for Canada, France, the UK and the USA). This model reveals how rare, but large, interurban migratory shocks dominate city growth, and that the distribution of city populations have a complex shape that is not described by Zipf’s law.
Bio: Marc Barthelemy is a Research Director at the Institute of theoretical physics (IPhT) in Saclay (France) and an associate member of the Center for Social Analysis and Mathematics (CAMS/EHESS). He worked on applications of statistical physics to complex systems, complex networks, theoretical epidemiology, and spatial networks. Focusing on both data analysis and modeling, Marc is currently working on various aspects of urban systems.
Website: www.quanturb.com
25 Nov 2021 – Renaud Lambiotte (Oxford) – Order and Disorder in Network Science
Abstract: A recurring theme in the study of complex systems is the emergence of order and disorder in systems. Historically, one can think of the Boltzmann equation, and the irreversible growth of disorder at the macroscopic scale from reversible dynamics at the microscopic scale. Reversely, scientists have been fascinated by the emergence of spatial and temporal patterns in interacting systems. In this talk, I will give a personal view on these two sides within the field of network science, whose combination of order and randomness is at the core of several works on network dynamics and algorithms.
Bio: Renaud Lambiotte has a PhD in physics from the Université libre de Bruxelles. After postdocs at ENS Lyon, Université de Liège, UCLouvain and Imperial College London, and a professorship in Mathematics at the University of Namur, he is currently associate professor at the Mathematical Institute of Oxford University. His main research interests are the modelling and analysis of processes taking place on large networks, with a particular focus on social and brain networks.
16 Sept 2021 – Laura Cantini (CNRS, ENS) – Network-guided integration of multi-omics data: towards a comprehensive view of cancer
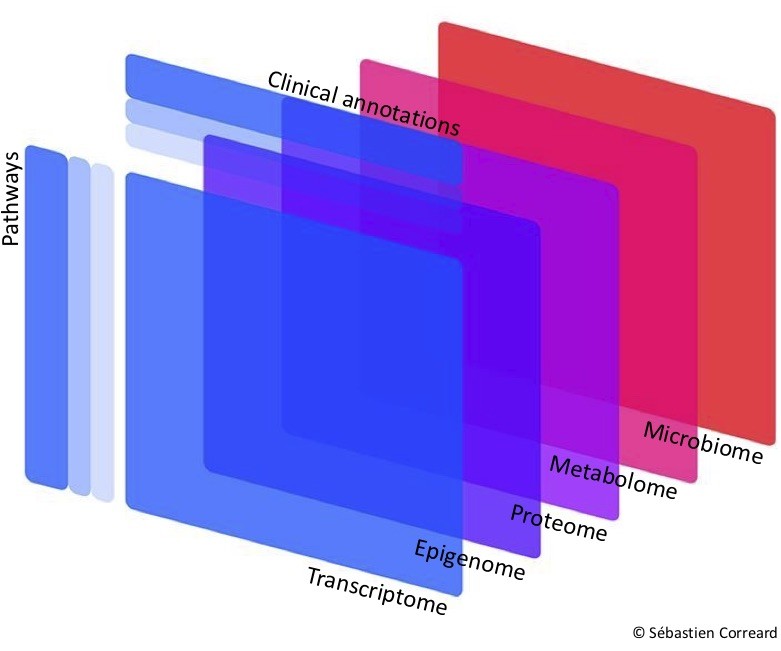
Abstract: Due to the advent of high-throughput technologies, high-dimensional “omics” data are produced at an increasing pace. In cancer biology, national and international consortia have profiled thousands of tumors at multiple molecular levels (“multi-omics”) allowing to gather a comprehensive molecular picture of this disease. Moreover, multi-omics profiling approaches are currently being transposed at single-cell resolution, further increasing the information accessible from cancer samples. The current main challenge is to design appropriate methods to integrate this wealth of information and translate it into actionable biological knowledge. In this talk I will give some examples on the use of networks to mine and integrate multi-omics data with the ultimate aim of revealing new insights into cancer biology.
Bio: Laura Cantini is a CNRS permanent researcher at the Institut de Biologie de l’Ecole Normale Supérieure (IBENS). She develops machine learning methods for the integration of bulk and single-cell data. In addition, she closely collaborates with biologists to address specific biological questions by applying the developed methods. Mathematician by training, Laura received her PhD in cancer systems biology from the University of Turin (Italy). She then pursed a postdoc in the cancer system biology group at Institut Curie (Paris). In 2018, awarded of the L’Oréal-UNESCO for Women in Science and EMBO fellowship, she joined CSAIL at MIT (USA), before being selected as CNRS permanent researcher. In 2020, She is recipient of an ANR JCJC and Sanofi iTech Awards funding which gave her the possibility to start her independent research line.
8 July 2021 – Marc Timme (TU Dresden) – Inverse problems and data-driven modeling for multi-dimensional dynamical systems — from biology to mobility
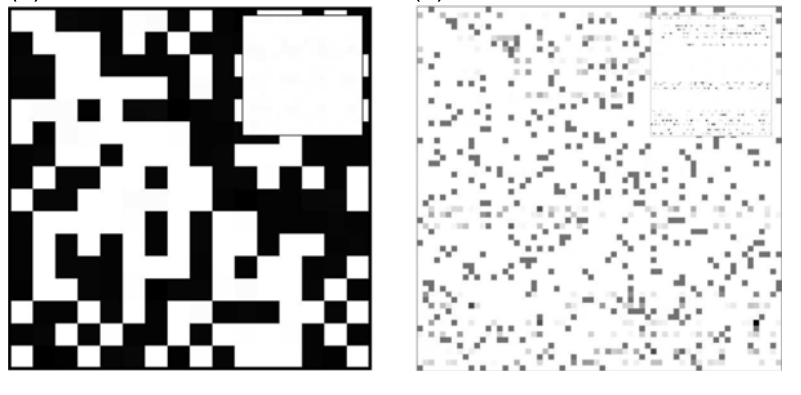
Abstract: Inverse problems have a long history in the natural sciences and mathematics and are implicit to many engineering problems. For instance, Bragg diffraction techniques yield lattice spacings of periodic crystal lattices and design and closed-loop control schemes enable the reliable functioning of engineered machines near set operating points. Yet, for multi-dimensional systems the vast majority of research on collective nonlinear dynamics is still focusing on the “forward direction” of analysis or modeling and asks what types of collective dynamics emerge from a network of given units interacting via a given topology. How to infer features of systems that both are multi-dimensional and exhibit self-organized dynamics thus remains an open problem. Here we address two basic questions on network inference. First, how to identify the number of dynamical variables in a multi-dimensional system from observing time series of only some of them? Second, how to infer interaction topologies from recorded time series only, in particular without the knowledge of any specific model of the system? Both constitute partially uncharted territory for theory and may offer a wide range of applications. We also touch the dynamics of multi-dimensional mobility systems that pose even harder inference problems due to little data available and simultaneously complex, externally driven dynamics.
Example References:
[1] Theory of topology inference: Science Adv. 3: e1600396 (2017) and Nature Comm. 8:2192 (2017).
[2] Theory of system size inference: Phys. Rev. Lett. 122:158301 (2018).
[3] Constraining features of mobility systems: Nature Comm. 11: 4831 (2020) and Nature Comm. 12: 3003 (2021).
Bio: Marc studied applied mathematics and physics in Würzburg (Germany), Stony Brook (New York, USA), and Göttingen (Germany) and holds a doctorate in Theoretical Physics. After working as a Postdoctoral Researcher with the Max Planck Institute for Flow Research and as a Research Scholar at Cornell University, Ithaca, New York, USA, he was selected to head a broadly cross-disciplinary Max Planck Research Group on Network Dynamics at the Max Planck Institute for Dynamics and Self-Organization. He held a Visiting Professorship at TU Darmstadt and was a Visiting Faculty at ETH Zurich. Since 2018, he has been an Honorary Member of Lakeside Labs, Klagenfurt. He is currently a Strategic Professor and the Head of the Chair of Network Dynamics at the Cluster of Excellence Center for Advancing Electronics Dresden (cfaed) and the Institute for Theoretical Physics, TU Dresden. He is also the Co-Chair of the Division of Socio-Economic Physics of the German Physical Society (DPG). His research integrates first principles theory with data-driven modeling to establish generic fundamental insights that drive
applications of complex dynamical systems, including bio-inspired information processing, energy systems, collective mobility and transport, as well as systemic sustainability.
3 June 2021 – Gerardo Iñiguez (CEU) – Dynamics of cascades on burstiness-controlled temporal networks

Abstract: Burstiness, the tendency of interaction events to be heterogeneously distributed in time, is critical to information diffusion in physical and social systems. However, an analytical framework capturing the effect of burstiness on generic dynamics is lacking. Here we develop a master equation formalism to study cascades on temporal networks with burstiness modelled by renewal processes. Supported by numerical and data-driven simulations, we describe the interplay between heterogeneous temporal interactions and models of threshold-driven and epidemic spreading. We find that increasing interevent time variance can both accelerate and decelerate spreading for threshold models, but can only decelerate epidemic spreading. When accounting for the skewness of different interevent time distributions, spreading times collapse onto a universal curve. Our framework uncovers a deep yet subtle connection between generic diffusion mechanisms and underlying temporal network structures that impacts a broad class of networked phenomena, from spin interactions to epidemic contagion and language dynamics.
Unicomb, S. Iñiguez, G., Gleeson, J. P., Karsai, M. “Dynamics of cascades on burstiness-controlled temporal networks.” Nature communications 12.1 (2021): 1-10. https://doi.org/10.1038/s41467-020-20398-4
Bio: Gerardo Iñiguez is assistant professor at the Department of Network and Data Science of Central European University (CEU, Austria), visiting researcher at Aalto University (Finland) & UNAM (Mexico), and CEO & co-founder at Predify (Mexico), a data science consulting startup. He’s also Academic Editor at PLoS ONE and Information, member of the European Humane AI Net project, and member of the Data Analytics Faculty Group of the Executive MBA program at CEU. Gerardo’s research involves the empirical analysis and mathematical modeling of dynamics of/on networks, including analytical formalisms to describe network dynamics. He’s worked in information and behavioral spreading over techno-social networks, in the dynamics of rank and hierarchies in complex systems, in conflict emergence and resolution in online collaborative environments, in opinion formation and deception in adaptive social networks, and in the sustainability of urban mobility. Gerardo also writes fiction, trains martial arts, and co-develops video games in Salmon Snake Studio, an indie developer in Mexico. Find out more on his website at: http://www.gerardoiniguez.com.
21 Jan 2021 – Jürgen Kurths (Humboldt University) – Quantifying Stability in Deterministic and Stochastic Complex Networks and its Application to Power Grids
Abstract: Power grids, the human brain, arrays of coupled lasers, genetic networks, or the Amazon rainforest are all characterized by multistability. The likelihood that these systems will remain in the most desirable of their many stable states depends on their stability against significant perturbations, particularly in a state space populated by undesirable states. Here we claim that the traditional linearization-based approach to stability is in several cases too local to adequately assess how stable a state is. Instead, we quantify it in terms of basin stability, a new measure related to the volume of the basin of attraction. Basin stability is non-local, nonlinear and easily applicable, even to high-dimensional systems. It provides a long-sought-after explanation for the surprisingly regular topologies of neural networks and power grids, which have eluded theoretical description based solely on linear stability. Remarkably, when taking physical losses in the network into account, the back-reaction of the network induces new exotic solitary states in the individual actors, and the stability characteristics of the synchronous state are dramatically altered. These novel effects will have to be explicitly taken into account in the design of future power grids, and their existence poses a challenge for control.
Bio: Jürgen Kurths studied mathematics at the University of Rostock. He received the Ph.D.degree from the GDR Academy of Sciences, in 1983. He was a Full Professor with the University of Potsdam, from 1994 to 2008. He has been a Professor of nonlinear dynamics at the Humboldt University, Berlin, and the Chair of the Research Domain Complexity Science of the Potsdam Institute for Climate Impact Research, since 2008. He has published more than 600 articles that are cited more than 48,000 times (H-factor: 99). His primary research interests include synchronization, complex networks, and time series analysis and their applications in Earth Sciences, Physiology, infrastructure and others. He is a Fellow of the American Physical Society. He became a member of the Academia Europaea, in 2010. He received the Alexander von Humboldt Research Award from CSIR, India, in 2005, and the Richardson award from the European Geoscience Union in 2013. He got eight Honory Doctorates and Honorary Professors. He is Editor-in-chief of the Journal of Nonlinear Science, and Chaos and editor of about 10 further journals, such as Europ. Physics Lett.
References:
P. Menck, J. Heitzig, N. Marwan, and J. Kurths, Nature Physics 9, 89 (2013)
P. Menck, J. Heitzig, J. Kurths, and H. Schellnhuber, Nature Communication 5, 3969 (2014)
Y. Zou, T. Pereira, M. Small, Z. Liu, and J. Kurths, Phys. Rev. Lett. 112, 114102 (2014)
F. Hellmann, P. Schultz, C. Grabow, J. Heitzig, and J. Kurths, Scient. Rep. 6, 29654 (2016)
Y. Xu, Y. Li, H. Zhang, X. Li, and J. Kurths, Scient. Rep. 6, 31505 (2016)
J. Nitzbon, P. Schultz, J. Heitzig, J. Kurths, and F. Hellmann, New J. Phys. 19, 033028 (2017)
F. Hellmann, P. Schultz, P. Jaros, R. Levchenko, T. Kapitaniak, J. Kurths, and Y. Maistrenko, Nature Communications 11, 592 (2020)
10 Dec 2020 – Tiago P. Peixoto (CEU) – Network reconstruction from indirect observations
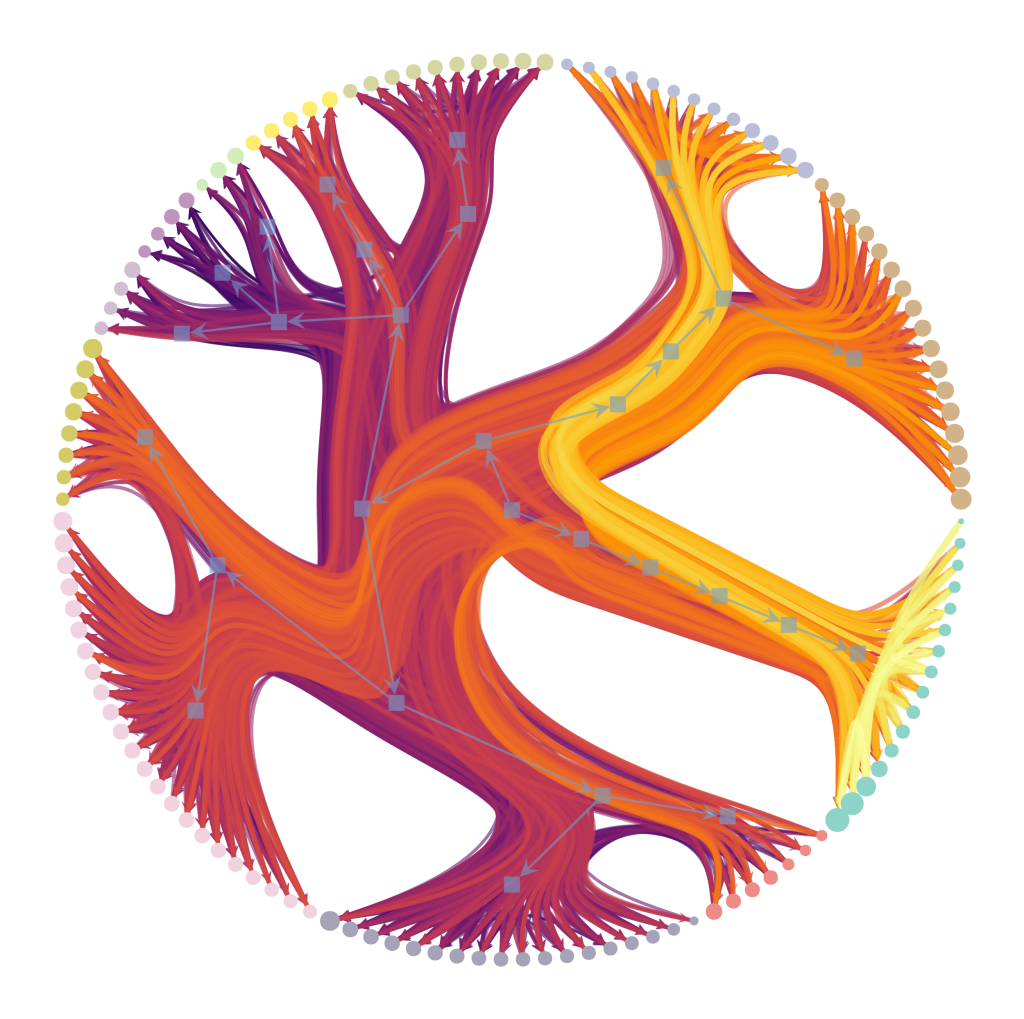
Abstract: The observed functional behavior of a wide variety of large-scale systems is often the result of a network of pairwise interactions. However, in many cases these interactions are hidden from us, either because they are impossible or very costly to be measured directly, or, in the best case, are measured with some degree of uncertainty. In such situations, we are required to infer the network of interactions from indirect information.
In this talk, I present a scalable Bayesian method to perform network reconstruction from indirect data, including noisy measurements and observed network dynamics. This kind of approach allows us to convey in a principled manner the uncertainty present in the measurement, and combined with versatile modelling assumptions can yield good results even when data are scarce. In particular, I describe how the reconstruction approach can be combined with community detection, allowing us to tap into multiple sources of evidence available for the task. I show how this combined approach provides a twofold improvement, by increasing not only the reconstruction accuracy, but also the identification of communities in networks. The latter improvement is possible even in situations where at first we might imagine that reconstruction is superfluous, for example when direct network data are available and measurement errors can be neglected.
Bio: Tiago P. Peixoto is an Associate Professor at the Department of Network and Data Science at the Central European University (https://networkdatascience.ceu.edu/people/tiago-peixoto). His research focuses on characterizing, identifying and explaining large-scale patterns found in the structure and function of complex network systems — representing diverse phenomena with physical, biological, technological, or social origins — using principled approaches from statistical physics, nonlinear dynamics and Bayesian inference. Peixoto develops and maintains graph-tool — an efficient Python module for manipulation and statistical analysis of graphs and networks. He has a PhD in Physics from the University of São Paulo, and a Habilitation in Theoretical Physics from the University of Bremen. In 2019 he was the recipient of the Erdős–Rényi Prize in Network Science, awarded by the Network Science Society.
19 Nov 2020 – Michele Starnini (ISI Foundation) – Emergence of echo chambers and polarization dynamics in social networks
Abstract: Echo chambers and opinion polarization, recently quantified in several sociopolitical contexts and across different social media, raise concerns on their potential impact on the spread of misinformation and on the openness of debates. Despite increasing efforts, the dynamics leading to the emergence of these phenomena stay unclear. In this talk, we will first review empirical evidence for the presence of echo chambers across social media platforms, by performing a comparative analysis among Gab, Facebook, Reddit, and Twitter. Then, we will present a simple modeling framework able to reproduce the observed opinion segregation in the social network. We consider networked agents characterized by heterogeneous activities and homophily, whose opinions can be reinforced by interactions with like-minded peers. We show that the transition between a global consensus and emerging polarized states in the network can be analytically characterized as a function of the social influence of the agents and the controversialness of the topic discussed. Finally, we consider a generalization to multiple opinions with respect to different topics. Inspired by skew coordinate systems recently proposed in natural language processing models, we frame this problem in a formalism in which opinions evolve in a multidimensional space where topics form a non-orthogonal basis. We show that this approach can reproduce the correlations between extreme opinions on different topics found in survey data.
Bio: Michele Starnini is currently Research Fellow in Network and Data Science at ISI Foundation. Before, he was Principal Investigator of a project funded by the J. S. McDonnell Foundation, at the University of Barcelona. His interests are the understanding of emerging socio-economic phenomena, such as the epidemic spreading of behaviors or ideas in a population, the dynamics of physical interactions in social gatherings, or cooperation/collaboration among individuals. At a more theoretical level, he studies the behavior of dynamical processes on static and time-varying networks.
12 Nov 2020 – Geoffroy Berthelot (INSEP) – Pseudo-Darwinian network evolution
Abstract:
The paper which we will present you is from our recent publications [1,2] and is related to the topic of extreme cost optimization and network fragility/vulnerability.
Variety of natural and human-engineered processes including biological, societal and technological ones can be presented as transportation networks.
Common examples include blood vessel networks and the lung airway tree that deliver blood and oxygen molecules.
In our work the evolution of complex transport networks is investigated under three strategies of link removal: random, intentional attack and “Pseudo-Darwinian” strategy. At each evolution step and regarding the selected strategy, one removes either a randomly chosen link, or the link carrying the strongest flux, or the link with the weakest flux, respectively. We study how the network structure and the total flux between randomly chosen source and drain nodes evolve. We discover a universal power- law decrease of the total flux, followed by an abrupt transport collapse. The time of collapse is shown to be determined by the average number of links per node in the initial network, highlighting the importance of this network property for ensuring safe and robust transport against random failures, intentional attacks and maintenance cost optimizations
5 Nov 2020 – Massimo Stella (University of Exeter) – Cognitive network science as a proxy for accessing key ideas and emotions in people’s minds
Abstract: This talk focuses on recent applications of network science to the quantification of cognitive patterns about people’s perceptions. The recent field of cognitive network science, at the intersection between computer science, psycholinguistics and complex systems, provides novel tools for reconstructing how people frame and perceive events by means of conceptual associations, sentiment analysis and emotional profiling. Different case studies will be investigated, such as: (i) identifying subjective perceptions of STEM subjects in students and researchers, (ii) reconstructing the emotional profiles of social discourse around the gender gap in science and (iii) the identification of emotional patterns over time within the social discourse of COVID-19. Research gaps and potential bridges with natural language processing and AI will be highlighted, as well as theoretical motivations brought up by the current literature in cognitive science. Cognitive networks provide a transparent access to knowledge structure and its perceptions, opening the way to important future applications for policy making, interpretable AIs and social good.
8 Oct 2020 – Elisa Omodei (World Food Programme’s Research) – Who, where, why: non-traditional data and predictive analytics to map socio-economic vulnerabilities

Abstract: In a rapidly changing world, severely affected by extreme weather events, epidemic outbreaks, economic shocks and conflicts, it is of fundamental importance to understand where the most vulnerable people are, how many they are, and to identify what it is that makes them more vulnerable than others to these threats. During the last decade, research has shown that data such as digital traces, phone metadata and satellite imagery carry relevant information beyond their original purpose and can be used as a proxy to measure socio-economic characteristics and detect vulnerabilities when traditional data is not available. Following an overview of these studies, the talk will deep dive into the UN World Food Programme’s original work on predicting food security. We will then conclude by discussing challenges and limitations, but also opportunities, that come with these approaches.
Bio: Elisa Omodei is the Predictive Analytics Lead of the Hunger Monitoring Unit at the World Food Programme’s Research, Assessment and Monitoring division. She also serves as Vice-President Secretary of the Complex Systems Society. She holds a BSc and a MSc in Physics and a PhD in Applied Mathematics for the Social Sciences. After her PhD, she spent a few years in academia before joining the United Nations in 2017, first at UNICEF’s Office of Innovation and now at the World Food Programme.
9 July 2020 – Petter Holme (Tokyo Tech) – Freedom of choice adds value to public goods
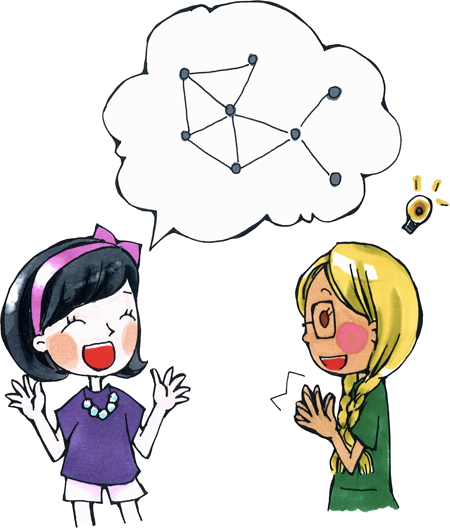
Abstract: Public goods, ranging from judiciary to sanitation to parkland, permeate daily life. They have been a subject of intense interdisciplinary study, with a traditional focus being on participation levels in isolated public goods games (PGGs) as opposed to a more recent focus on participation in PGGs embedded into complex social networks. We merged the two perspectives by arranging voluntary participants into one of three network configurations, upon which volunteers played a number of iterated PGGs within their network neighborhood. The purpose was to test whether the topology of social networks or a freedom to express preferences for some local public goods over others affect participation. The results show that changes in social networks are of little consequence, yet volunteers significantly increase participation when they freely express preferences. Surprisingly, the increase in participation happens from the very beginning of the game experiment, before any information about how others play can be gathered. Such information does get used later in the game as volunteers seek to correlate contributions with higher returns, thus adding significant value to public goods overall. These results are ascribable to a small number of behavioral phenotypes, and suggest that societies may be better off with bottom-up schemes for public goods provision.
28 May 2020 – Luca Aiello (Bell Labs, Cambridge) – Ten Dimensions of Social Relationships
Abstract: Social relationships are the key determinant of crucial societal outcomes, including diffusion of innovation, productivity, happiness, and life expectancy. To better attain such outcomes at scale, it is therefore paramount to have technologies that can effectively capture the type of social relationships from digital data. NLP researchers have tried to do so from conversational text but mostly focusing on sentiment or topic mining, techniques that fall short on either conciseness or exhaustiveness. We propose a theoretical model of 10 dimensions of social relationships that is backed by decades of research in social sciences and that captures most of common relationship types. We trained a deep-learning model to classify text along these ten dimensions, and we reached performance up to 0.98 AUC. By applying this tool on large-scale conversational data, we show that the combination of the predicted dimensions suggests both the types of relationships people entertain and the types of real-world communities they shape. As a use case, we show how this technology can be used in the domain of employer care by predicting Nokia employee satisfaction from online review text
Bio: Luca Maria Aiello is a Senior Research Scientist in the Social Dynamics team at Nokia Bell Labs Cambridge, UK. Formerly, he has been a Research Scientist at Yahoo Labs for almost 5 years and a research Fellow of the ISI Foundation in Torino, Italy. He conducts interdisciplinary research in computational social science and network science. His work has been covered by hundreds of news articles published by news outlets worldwide including Wired, WSJ, and BBC. He is currently working on NLP research to model fundamental aspects of human relationships from conversational text.
27 Feb 2020 – Jean-philippe Cointet (Sciences Po Media Lab) – The structure of the French media space and the Yellow vest movement

Abstract: I will start by outlining the main results of the structural description of the French media sphere we are working on at médialab. We are collecting data at two distinct levels of the media system: the daily publication of articles by the 400 main news outlets, and the interactions that these articles incite (citations to media sources) on Twitter. The morphology of the media space that is emerging is characterized by a very high concentration of authority (particularly visible in the distribution of hypertext links) and a rather limited ideological polarization. This first result is in stark contrast with the structure of the media space as observed in the United States. We will finally consider how this organization is being challenged at another layer of the public space: conversations on Facebook. We will show, in particular, how Yellow Vests group pages redistribute order between the different media.
Bio: Jean-Philippe Cointet is working at the Sciences Po médialab, where he designs innovative computational sociology methods. He is specialized in text analysis, and is working on various kinds of corpora and sources questioning their socio-political dynamics. His research areas are diverse, ranging from social media analysis (Facebook public posts and comments) to science of science (data turn in oncology)or political processes mapping (political discourses, international negotiations) and frame analysis (press coverage of migration processes). He also participates in developing the CorText platform. He holds a PhD in Complex Systems and was trained as an engineer at Ecole Polytechnique. He is also affiliated with the research center INCITE, from Columbia University.
28 Nov 2019 – Blaise Delmotte (CNRS, LadHyX, Ecole Polytechnique) – Leveraging Collective Effects in Active Fluids

Abstract: We have all witnessed the flocking of starlings in the sky and the schools of fish that form in the ocean. This kind of organization of living systems is not limited to those that we see, but also occurs for those that we don’t: swimming micro-organisms. Collections of micro-swimmers, such as bacteria or sperm cells, exhibit a rich dynamics. They can form coherent structures due to collective motion, mix the surrounding fluid or tune some of its effective physical properties. These fascinating phenomena raise a fundamental question: how can a living system self-organize without any sensorial or cognitive functions ?
Beyond the fundamental interest in the physical understanding of such “active fluids”, their precise modeling is also likely to have applications to the design of new fluid systems with synthetic controllable properties. More recently, artificial micro-swimmers have been developed to mimic these behaviors, but also to achieve specific functions, such as targeted drug delivery or transport in microfluidic systems.
Active matter therefore offers promising perspectives for the design of smart meta-materials, but also for miniaturized and medical technologies.
In this talk, I will show how to combine experiments, numerical modeling and theoretical calculations to explore different ways to use and control biologic and synthetic micro-swimmers for self-assembly, enhanced fluid mixing and particle transport at the microscopic level.
17 Oct 2019 – Brennan Klein (Network Science Institute, Northeastern University) – The structure is the story: How the right representation can bring forth new theories in complex systems

The effectiveness of a network at modeling a given system is intrinsically linked to its ability to express potential or counterfactual interactions in the system. If these neurons fired, would you observe a certain behavior? If I start a rumor, would you hear about it? This ability to encode counterfactual information in their structure makes complex networks rich philosophical objects as well. In this talk, I explore the role—and power—of representation in science and, in particular, complex systems science. To do this, I will primarily focus primarily on two recent projects about representation in network science. First, I will describe a general framework for coarse-graining complex networks to their most informative scale, a phenomenon known as causal emergence. Second, I will describe recent work that compares dozens of tools that are commonly used to infer network structure from time series data. This project is the culmination of a months-long “Collabathon” based out of the Network Science Institute at Northeastern University, and it features contributions from 33 different network scientists from around the world. I will close by briefly describing the structure of this style of research, encouraging more of these large, rigorous, and fun collaborations.
Bio: Brennan is a fifth-year PhD student studying surprise in complex systems. He is focused on understanding how complex systems are able to represent, predict, and intervene on their surroundings across a number of different scales—all in ways that minimize the surprisal experienced in the future. This approach is used to study a range of phenomena from decision making, to experimental design, to causation and emergence in networks. He is currently working with Alessandro Vespignani on a dissertation examining the teleology of networks, or why there appears to be an apparent purpose or goal-directedness to the dynamics and structure of networks. Brennan received his BA in Cognitive Science and Psychology from Swarthmore College in 2014, where he studied the relationship between perception, action, and cognition. He makes art under the pseudonym JK Rofling.
9 Oct 2019 – Ray Rivers (Imperial College) – Myth and Math: A network approach to Atlantis
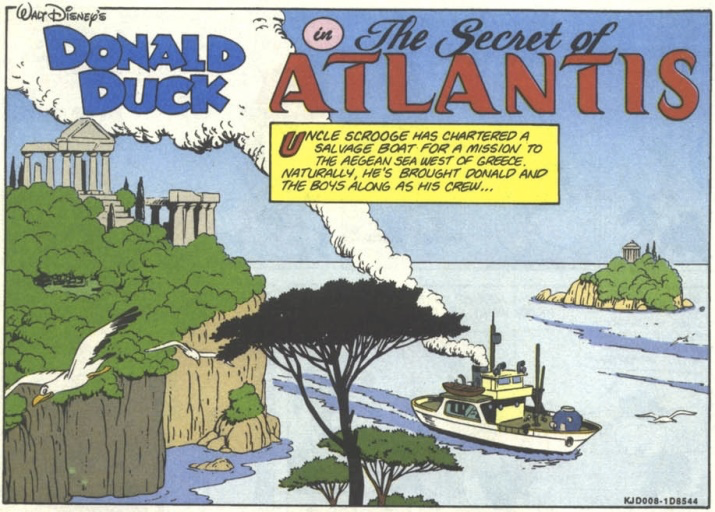
The Aegean archaeologist Carl Knappett, Tim Evans and myself have used network models to try to understand the effect of the eruption of Thera on the demise of the MBA Minoan thalassocracy. The destruction of Thera is often taken to be the basis for the Atlantis legend in popular culture (see D.Duck). I have been fascinated (and appalled) by the continuing potency of this myth, particularly with the European Alt-Right (Google ‘aryan atlantis’ to see). In this talk I will argue that disaster myths have their contradictions and reconsider Thera. I shall also briefly put this network modelling in the context of networking of text-based myths and stories.
Bio: Ray Rivers is Emeritus Professor in Theoretical Physics and a member of The Centre for Complexity Science at Imperial College London. However, in addition to continuing to work on fundamental quantum physics, for the last decade and more he has been active in archaeological network modelling, particularly of the Bronze Age Aegean. Most recently he is concerned with more epistemic issues such as how we impose the present on the past.
3 Oct 2019 – Elizabeth Vergu (INRA) – Data analysis and model-based indicators of nodes criticality for epidemic spread and control on a cattle trade network
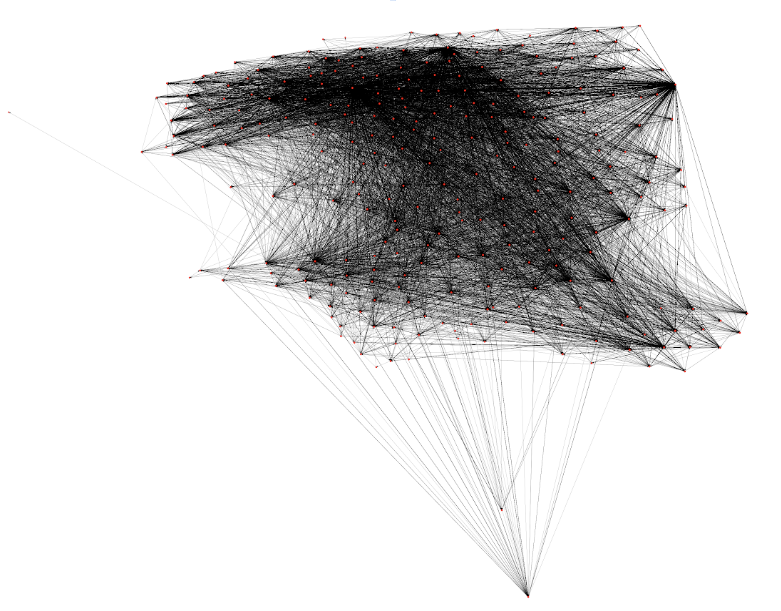
Informing prevention and control of infectious diseases in livestock populations at regional level necessitates the investigation of the underlying structure of spread represented by animal trade network and the coupling of intra-herd infection dynamics. Despite an abundant literature about dynamical epidemics on contact networks, generic tools are still needed when nodes are subpopulations, to characterize the impact on the infection dynamics of the interplay between network structure and local demography.
In this talk, I will first show some results from the analysis of the French cattle movement network over several years to investigate the fidelity over time of transaction partners. Proxies for pathogen spread, such as reachability ratio, accounting for network time-varying properties, were also computed, using efficient algorithms of network exploration, to assess spread and control of epidemics for various assumptions. In the second part, I will present theoretical results based on a model coupling metapopulation and epidemic dynamics on an explicit network and applied to the French data on cattle trade to build and assess graph vulnerability indicators and explore control strategies.
26 Sept 2019 – Vincent Hakim (CNRS, ENS) – Puzzles about long term memory and neuronal learning
Synapses are important biological structures that serve to transmit information between neurons and are thought to be the sites of learning and memory. Yet, it has remained enigmatic how memory can be retained for years while synaptic components turnover over the course of hours. Similarly, when learning a complex task, the received feedback seems most often global and poorly informative. It is then quite unclear how the strengths of different synapses belonging to numerous different neurons can be properly adjusted, the so called « credit assignment problem ». After recalling some element of synapse biophysics and relevant experimental findings, I will discuss our recent work in collaboration with the teams of A Triller and B Barbour at IBENS, aiming at addressing these two puzzles.
Bio: Vincent Hakim holds a CNRS Research Director position at Ecole Normale Supérieure (ENS) in Paris, France. He is a theoretical physicist specialised in statistical physics and nonlinear dynamics. During the last twenty years, he has carried out theoretical work in theoretical neuroscience and developmental biology, much of it in close collaboration with experimental teams.
12 Sept 2019 – Federico Battiston (CEU) – New emergent behavior in generalized networks

Networks constitute the backbone of many complex systems, on top of which collective behavior can emerge: from epileptic seizures in the human brain to the viral spread of rumours in a social network. When are simple network representations not enough? In this talk I introduce the topic of generalized networks, richer architectures which allow to consider the temporal and multiplex dimensions of relationships, and to go beyond simple pairwise interactions. Building on my contributions to the field, I focus on how to measure multiplexity in real-world systems from the micro to the macro scale, with examples from social, transportation and biological networks. I will then provide a short perspective on dynamical processes on generalized networks, searching for new emergent collective behavior and warning against overly optimistic statements associated to the multiplex, temporal and non-dyadic nature of interactions.
Bio: Federico is an Assistant Professor at the Department of Network and Data Science at Central European University. Federico studies the structure and the dynamics of complex systems, using his background as statistical physicist (B.Sc. and M.Sc. from Sapienza University of Rome) to look into biological problems, model social systems, and find new solutions for the design of man-made networks. He holds a PhD in Applied Mathematics from Queen Mary University of London, where he was a member of the Complex Systems and Networks Group and worked under the supervision of Vito Latora as part of the EU-FP7 project LASAGNE on multilayer networks. Federico is an elected member of the council of the Complex Systems Society and a former Chair of the Young Researchers of the Society. Before joining DNDS, Federico held postdoctoral positions at the Brain & Spine Institute in Paris, and at the Department of Anthropology at University College London.
14 July 2019 – Jennifer Dunne (Santa Fe Institute) – Examining human-centered ecological networks across the world

Abstract: Some of our best opportunities for generalizing and enhancing ecological understanding come from studies of ancient ecosystems. One frontier of such research focuses on ecological networks. In this talk, I’ll take a deep-time perspective on food web structure and dynamics. First I’ll briefly discuss the organization of food webs from ecosystems 100s to 10s of millions of years old. Second I’ll discuss a new research agenda to study archaeo-ecological networks from the last several thousand years that include pre-industrial humans. These types of deep-time data sets and analyses provide important perspectives on ecosystem stability, robustness and sustainability as we move further into the Anthropocene.
Bio: Jennifer Dunne is the Vice President for Science at the Santa Fe Institute, where she has been on the faculty since 2007. Dunne has degrees in philosophy (A.B., Harvard), ecology and systematic biology (M.A., San Francisco State University), and energy and resources (Ph.D., University of California, Berkeley). Her research interests are in analysis, modeling and theory related to the organization, dynamics, stability and function of ecological networks at multiple spatial and temporal scales. Dunne was named a Fellow of the Ecological Society of America in 2017. Her publications have appeared in journals such as Proceedings of the National Academy of Sciences USA, Trends in Ecology and Evolution, PLoS Biology, Philosophical Transactions of the Royal Society B, Proceedings of the Royal Society B, Ecology Letters, Ecology, and Ecological Monographs. Media outlets including Scientific American, Wired, SmartPlanet, ScienceNow, Nature News, and Quanta have reported on Dunne’s research.
27 June 2019 – Daniele Marinazzo (Ghent University, Belgium) – Synergy and Redundancy: a Network Perspective
Synergy and redundancy are ubiquitous yet elusive concepts. Frameworks rooted in information theory allow to quantitatively define them, in particular quantifying the joint information shared by two drivers on a target. In this contribution, we will add networks to the picture in three aspects:
1. Synergy and redundancy in building networks: higher order interactions are often ignored when computing dynamical interactions in multivariate systems. I will try to convince you that this is both necessary and informative.
2. Synergy and redundancy as networks: defining a pairwise synergy index we can define networks in which the nodes are pairs of drivers, and the links determine whether they share joint information on a given target. The resulting network has a hierarchical structure, and we can see shared information mapped on graph theory quantities.
3. Synergy and redundancy between networks: we address the information transferred between networks, in a directed way, or mediated by another network. We will present a simple theory and two simple applications, to social media, and neural data.
Figure from The Neuron Doctrine [Cajal, 1894]
20 June 2019 – Charles Baroud (Ecole Polytechnique, Pasteur, France)

Abstract: In this presentation I will discuss our work on 3D cell culture in droplet microfluidics. I will begin by explaining the technique and how it allows us to make, manipulate, and observe hundreds spheroids or organoids in parallel. Then I will show some new biological insights we have gained by generating very large sets of single-cell data within model organoids. Finally, I will describe some recent work to understand the interactions between immune cells and cancer models, and how network theory can help us understand the biology of the 3D spheroids.
Bio: Charles Baroud studied physics and mechanical engineering at MIT then U. Texas at Austin. In 2001 he joined the Laboratoire de Physique Statistique at ENS as a post-doc, working on reaction-diffusion experiments. Since 2002 he has held a faculty position at Ecole Polytechnique, where he has worked on different problems in microfluidics, first covering the physical aspects and more recently working on biological applications. Since 2018 he has also led a research unit at Institut Pasteur, focusing on the use of droplet techniques to learn new biology.
13 June 2019 – Henry C. W. Price (Imperial College, London) – From Local to Global: Nested Interaction and Community in Late Bronze Age Crete. A network approach.

Abstract: Using as series of null models, we attempt to reconcile complex systems and the simple models that come to characterize much of the explained distribution of artifacts and settlement relationships to the wider Mediterranean. We also try and describe when these models are inappropriate.
Bio: Henry C. W. Price is currently a doctoral candidate in Theoretical Physics at Imperial College London. Working on a diverse number of topics in network theory and applied mathematics. Mainly Complexity Science, Complex Networks and Directed Acyclic Graphs. Actively researching Cryptocurrency and distributed ledger technology with University of London research groups and others bodies, he also works on machine learning and data science projects in Reg/Fin-tech. With an undergraduate degree in Theoretical Physics at Imperial. Henry also studied Mathematics and Financial Modelling and wrote his MSc thesis on Volatility. His most recent research also concerns computational methods in the digital humanities, social and life sciences, in addition to derivatives and futures contracts on Bitcoin.
6 June 2019 – Janet Bennion (Vermont University) – Polyamory in Paris: A Social Network Theory Application

Abstract: This research applies social network theory to the polyamory networks (polycules) of Paris, where participants in multiple loving relationships (both partners and metamours) share an exchange of information, ideas, and an assortment of valued prestations such as sexuality, friendship, and money. In such non-monogamous networks, there exists a vast web of nodes connected in much more intimate and complex ways than one finds in the mononormative landscape. This study seeks to evaluate the specific social capital exchanged by 45 individuals in the Polyamour/Polyamorie Paris Facebook group, assessing the strengths and weaknesses of their ties, and better understanding how they navigate the web to simultaneously live intimately with others and still fulfill their own selfish needs and desires.
Bio: Janet Bennion is a Professor of Anthropology from Northern Vermont University. She is the preeminent scholar on polygyny among Mormon Fundamentalist groups, having published extensively on female networking and the variability of poly lifestyles, including Women of Principle (University of Oxford Press, 1998) and Polygamy in Primetime (Brandeis University Press 2012). Her latest publication is collaborative and international, stemming from several international conference discussions about how to handle the poly world legally, compiled in the volume, The Polygamy Question (Utah State and Colorado Presses, 2015). Her most recent research explores polyamory networks in Paris where she is testing the efficacy of network theory on 45 subjects involved in 24 polycules.
23 May 2019 – Guillaume Dumas (Pasteur Institute) – Networks and Machine Learning in Biomedical Research: from Generative Models in Social Neurosciences to Multi-Scale Priors in Precision Medicine

Abstract: With the recent development of –omics fields, multiple sub-disciplines in life sciences have embraced networks as a valuable formalism. This presentation will present two projects illustrating how –omics networks can be used in biomedical research: 1) whole brain neuro-computational modelling with Connectomics, and 2) network-based stratification (NBS) with Genomics. The first example builds on the importance of anatomical and functional interactions in neuroscience; through modelling, numerical simulation provides insight into the basic mechanisms that enable integrative neural processes and how structural brain networks generate spatially and temporally organised brain activity at both intra- and inter-individual levels. The second example was originally designed for cancer research; the NBS combines genetic mutation profiles of patients with protein-protein interaction (PPI) networks to uncover clusters of patients with similar tumour subtypes. Here we will review the original results through a case study of reproducibility in bioinformatics and then explain the challenges to apply it to autism.
Bio: Guillaume Dumas is a researcher in the department of neuroscience of the Institut Pasteur in Paris. Originally from engineering and theoretical physics, he did a Ph.D. on cognitive neuroscience at the University of Paris 6 (UPMC) and then moved in a postdoc at the Center for Complex System and Brain Science of Florida Atlantic University. He came back to France for working in the “Human Genetics and Cognitive Functions” unit of the Institut Pasteur, where he started as a postdoc before receiving his permanent position. He then created the platform SoNeTAA (Social Neuroscience for Therapeutic Approaches of Autism) at the child and adolescent psychiatric department of the Robert Debré hospital in Paris. His interdisciplinary research is focused on integrative accounts of neural, behavioural and social coordination dynamics. Methods used range from both intra- and inter-individual neuroimaging techniques to neurocomputational simulations. He is also a science writer and journalist and is engaged with multiple projects at the cross-road of Art and Science. He has, for instance, co-founded HackYourPhD, a community advocating internationally the use of openness in Science and Knowledge as a common good, and ALIUS, an international and interdisciplinary research group dedicated to the investigation of the diversity of consciousness.
18 April 2019 – Alexey Medvedev (Université de Namur and Université catholique de Louvain) – Predicting discussion dynamics in online forums using Hawkes processes: case study of Reddit
Abstract: Online forums provide rich environments where users may post questions and comments about different topics. Understanding how people behave in online forums may shed light on the fundamental mechanisms by which collective thinking emerges in a group of individuals, but it has also important practical applications, for instance to improve user experience and increase engagement. Communication in online forums mostly happen through the open discussions, potentially available for all members of a particular platform. Cascading patterns, often emerging and well studied in online social media, are represented in forums in the form of threads, or, otherwise called, discussion trees. The two main questions arise: what is the shape and what is the dynamics of evolution of these trees? In this talk I will present an overview of the main research directions that arose in recent years and focus primarily on the most popular platform, Reddit. Then I will present a model of discussion trees generation based on the self-exciting Hawkes processes, which represents both the tree structure and temporal information. This model was applied to Reddit discussion trees and showed better accuracy in predicting future flow of the discussion, in comparison with the contemporary dynamic cascade models.
28 Feb 2019 – Chiara Poletto (INSERM) – Accounting for variable and heterogeneous human behaviour in the assessment of an epidemic
Abstract: Mathematical and computational approaches based on network theory and complex system dynamics are becoming increasingly effective in tackling open problems in infectious disease epidemiology. I will review my recent research work in this direction presenting studies on both fundamental problems and specific epidemic events. On the theoretical side, I will show how the structure and dynamics of human-to-human contacts alter the risk of an outbreak. This effect can be captured by the epidemic threshold, the critical transmissibility below which extinction is certain, that quantifies the vulnerability of a population to an infection. In order to compute this indicator we developed the infection propagator approach, where we used the multilayer framework to describe a temporal network and the Markov-chain formalism to solve the spreading dynamics in the critical regime close to the threshold. On the applicative side I will show how health seeking behavior, daily social contacts, and daily mobility patterns affect the initial transmission of an emerging disease and the propagation dynamics of recurrent epidemics at the city and regional level. These understandings were important for the epidemiological assessment of the MERS global dissemination, the recent outbreak of Zika and the seasonal influenza circulation.
Bio: Chiara Poletto is a researcher at the National Institute of Health and Medical Research (INSERM) in Paris (France), working on the spreading of infectious diseases seen as a complex system phenomenon. Epidemics are mediated by human contacts and, on a different scale, by human mobility patterns. Therefore, the role of network structure on infection risk, its persistence and impact on the population is a central question of her research work. Within this broad context, she is dedicating increasing attention to the physics of interacting spreading processes underlying important problems in disease ecology. Poletto received her PhD in Physics from the University of Padova (Italy) in 2009 and was then Post Doc at the Computational Epidemiology Laboratory, ISI Foundation, Torino (Italy), before joining the INSERM in 2012. She is recipient of the Junior Scientific Award of the Complex Systems Society for extraordinary scientific achievements, and of the starting grant from the program Emergence de la Ville de Paris. Her studies on emerging pathogens’ epidemics (from Ebola outbreak to Zika) have translated into expert advices for public health decision makers.
6 Dec 2018 – Christian Vestergaard (Pasteur Institute) – Randomized reference models for temporal networks
Abstract: Many complex systems can successfully be described as networks. This abstracts away most particularities of individual units in the system and allows to highlight and study the effects of the complex structure of their connections. Notable examples are human interaction networks and the spread of contagions and information in these, biological networks, including biotic interactions in ecosystems and neural connections in the brain, infrastructural networks, and world trade. The structure and dynamics of most empirically measured networks are complex and intrinsically correlated, making it particularly challenging to study them using traditional generative models.
As an alternative to the bottom-up approach of generative models, randomized reference models (RRMs) constitute a top-down approach to studying complex networks. RRMs deal with the controlled destruction of given temporal or topological structures in a complex network in order to create a distribution of reference networks retaining certain features of the original network. They are typically implemented as procedures that shuffle the edges of the network while retaining the features in question. This makes RRMs very generally applicable, and in particular in cases where we are not able to specify a realistic generative model. However, the effects of most shuffling procedures on network features remain poorly understood, complicating the interpretation of results, and the lack of a common framework has made it difficult to compare different RRMs.
I will describe a unified framework for the important class of RRMs generated by uniform shuffling procedures, which we by analogy to statistical physics will name microcanonical RRMs (MRRMs). Our framework lets us build a taxonomy of MRRMs that orders them and deduces their effects on important network features. It additionally tells us how we may generate new MRRMs by combining existing ones. I will discuss how MRRMs may be used as null models to identify statistically significant features in empirical networks. I will show how our framework can be used when we do not know how to choose the correct null model, and will apply this to characterize computational motifs in the Drosophila larval brain. I will finally show how series of different MRRMs may be used to pick apart the effects of different features of a network on dynamical processes unfolding on it.
Reference: https://arxiv.org/pdf/1806.04032.pdf
Bio: I am a statistical physicist and biophysicist with a main interest in data-driven modeling and in developing methods for the analysis of complex dynamical systems. The focus of my research has been on time-varying (temporal) social networks and single molecule dynamics in intracellular processes. I currently work on linking the physical structure and function of the brain to behavior.